AI: Black Swan and Echo Chambers: Future Risks and Scenarios
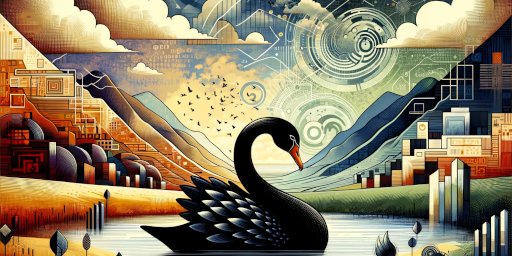
A very pressing and current question is circulating more and more often in talks on AI and future scenarios: what will happen to ChatGPT-{n} (but also to Bard, Mid Journey, Dall-E, Stable Diffusion, ….), once the Will large language models (*) help provide much of the language found online?
(*) A large language model (LLM Large Language Model) is a large-scale language model that has significant ability to achieve general-purpose language understanding and generation.
Generative AI allows the creation of texts and images that have invaded the web: the proliferation of this content, however, could create a short circuit on which researchers from different countries are focusing: the collapse model (see also: The Curse of Recursion: Training on Generated Data Makes Models Forget )
Model collapse is a degenerative process that affects generations of learned generative models, in which the generated data ends up polluting the training set of the next generation of models (which therefore incorrectly perceive reality by being trained on polluted data) .
This is a degenerative process in learning that risks self-perpetuating and consequently producing statistically more probable results and reducing less probable ones, producing a ‘flattening’ that enormously impoverishes the quality of content generation.
see also:
AI – Recursive Training of Generative Models
Generative ‘autophagy’ models are intended for MAD (Model Autophagy Disorder)
As highlighted in a recent study ( Self-Consuming Generative Models Go MAD ), without sufficient real data for each generation, future generative models are condemned to the so-called MAD (Model Autophagy Disorder), i.e. their quality (measured in terms of precision) or their diversity (measured in terms of recall) will progressively decrease and the degradation and generative artifacts will be amplified.
One doomsday scenario is that, if left unchecked for many generations, MAD could poison the data quality and diversity of the entire Internet.
Aside from that, it seems inevitable that hitherto invisible unintended consequences will result from AI autophagy even in the short term.
Another recent paper ( Nepotistically Trained Generative-AI Models Collapse ) highlights that “a diffusion-based text-to-image generative AI system is surprisingly vulnerable to data poisoning with its own creations.”
The importance of the black swan
Preserving the ability of LLMs to model low probability events is essential to the fairness of their predictions – even low probability events are vital to understanding complex systems [see the black swan theory: Nassim Nicholas Taleb – Black Swans and the Domains of Statistics ]
«… classic problem of induction: making bold claims about the unknown based on supposed properties of the known. So 1) the lower the probability, the larger the sample size needed to be able to make inferences, and the lower the probability, the larger the relative error in estimating this probability. 2) However in these domains, the lower the probability, the more consequential the impact of the absolute probability error on the moments of the distribution.» [Nassim Nicholas Taleb]
The issue of the ‘black swan’, a term coined by Nassim Nicholas Taleb to describe rare, unpredictable but impactful events, is particularly relevant in the context of the generative processes of artificial intelligence (AI). These systems, which learn from statistical distributions of data, may actually tend to overlook or underestimate the possibility of highly unlikely events, such as black swans.
Furthermore, just think of the greatest discoveries in science when they were made thanks to the contribution of true geniuses who were such in that they broke the mold and found new perspectives never thought of before (the exact opposite of the flattening in the degenerative process of learning) .
vedi anche AI – Il cigno nero: l’impatto dell’altamente improbabile
The echo chambers
Machine learning is widely used in product recommendation systems. Decisions made by these systems can influence users’ beliefs and preferences which in turn influence the feedback received from the learning system, thus creating a feedback loop.
This phenomenon can give rise to so-called ‘echo chambers’ or ‘filter bubbles’ which have implications for users and society.
The work is relevant in this sense: Degenerate Feedback Loops in Recommender Systems )
vedi anche:
AI – “camere dell’eco” e le “bolle di filtro”
Will competition help AI extricate itself from problems or amplify them?
The question is not just rhetorical: the strong competition in the scenarios introduced by AI pushes the main players to provide fast and immediate solutions, even if at the moment they may be at the expense of quality.
See the adoption, perhaps premature in some cases, of chatbots in search engines which on the one hand provide great advantages to users but on the other could amplify degenerative processes both in the generation of answers that do not adhere to reality and in the formulation of responses that tend to exclude unlikely events and conform to the general flatness.
Furthermore, even the users of AI services can contribute (even unconsciously) to the degeneration of the models, both because they use them without a critical sense and, perhaps out of laziness, because they want to force themselves to produce content based, at least essentially, on their own contribution.
It is certainly a challenge that will arise very often in future, if not already current, scenarios and it is very difficult to predict how it will develop, also considering that the explosion of AI at mass levels as seen today is only at the beginning and an exponential growth of developments and, alas, potential problems awaits us…
see also: La competizione tra i principali Player aiuterà l’AI a districarsi dai problemi o li amplificherà?